Sara Mathieson Awarded National Institutes of Health Grant
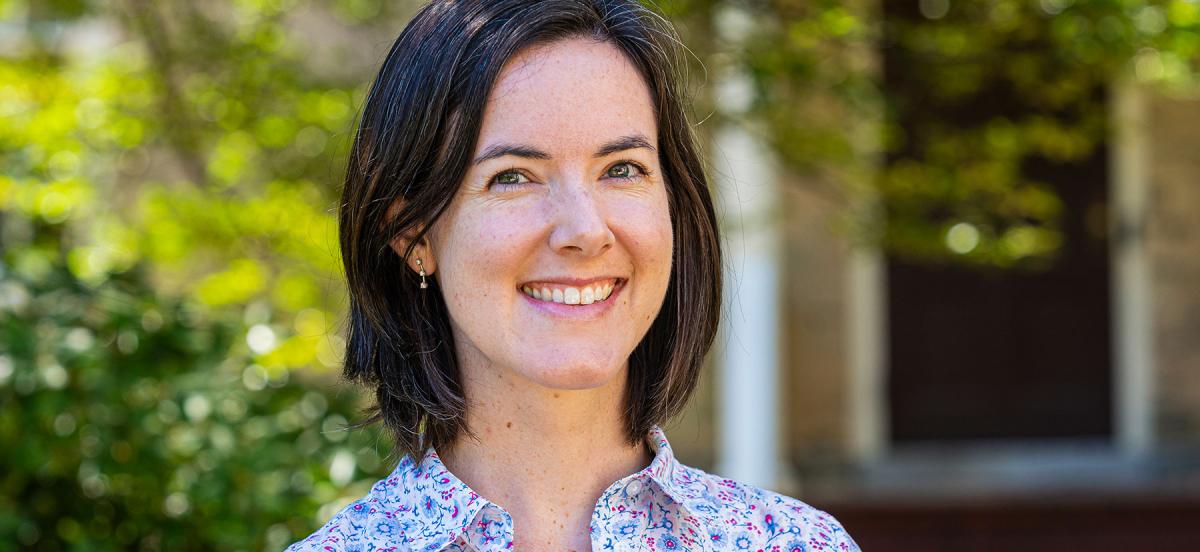
Sara Mathieson develops machine learning algorithms to understand evolution in humans and other species. Photo by Patrick Montero.
Details
The assistant professor of computer science earned the NIH award for a project that will use machine learning to develop algorithms for analyzing genomic datasets.
Sara Mathieson’s research lies at the intersection of computer science and biology. The assistant professor of computer science develops machine learning algorithms to understand evolution in humans and other species. A new grant from the National Institutes of Health will now support that work.
“Many of my long-term research goals involve using machine learning to make it easier to study new datasets without relying on a priori knowledge,” she said. “But the lack of training data has always been a limitation. That is why this project is so exciting, because it allows me to develop tools and approaches that will open up many new avenues of research, for me and other scientists.”
Mathieson notes that, over the past decade, scientists have sequenced hundreds of thousands of genomes—from humans as well as many other species—but that using this genomic data to answer biological questions requires new computational tools. Her NIH-funded project is one such tool that will use approaches from machine learning to develop algorithms to simulate necessary data.
Our methods will automatically adapt to diverse datasets, allowing us to quantify evolutionary forces in understudied human populations,” she said. “In addition, other researchers can use our pipelines to create simulated datasets that match their own population or species of interest. Downstream, the results will help us characterize the evolution of genetic variation that relates to human health, biodiversity, and environmental change.”
The NIH grant for $389,243 for her project, titled “Adaptive evolutionary inference frameworks for understudied populations using generative neural networks,” will be able to fund many research opportunities for Haverford students. Several Class of 2020 Fords—including Zhanpeng Wang, Jiaping Wang, Kendra Griesman, and Russell Gerhard—have already contributed to this work by either designing the prototype of the machine learning architecture or testing methods to separate out the impact of different evolutionary factors across human populations. Two current students, Trang Dang ’23 and Sam Tan ’23, are studying short-term evolution using pedigrees, which will also contribute to the proposed research.
Mathieson is excited that this work will help students see the connections between machine learning and the natural world, and she looks forward to the possible new collaborations across departments that it will engender.
“For me personally,” she said, “I am excited that the NIH sees the contributions of computer science and machine learning as powerful tools to answer biological questions.”