Team of Haverford Faculty and Students Publish in "Nature"
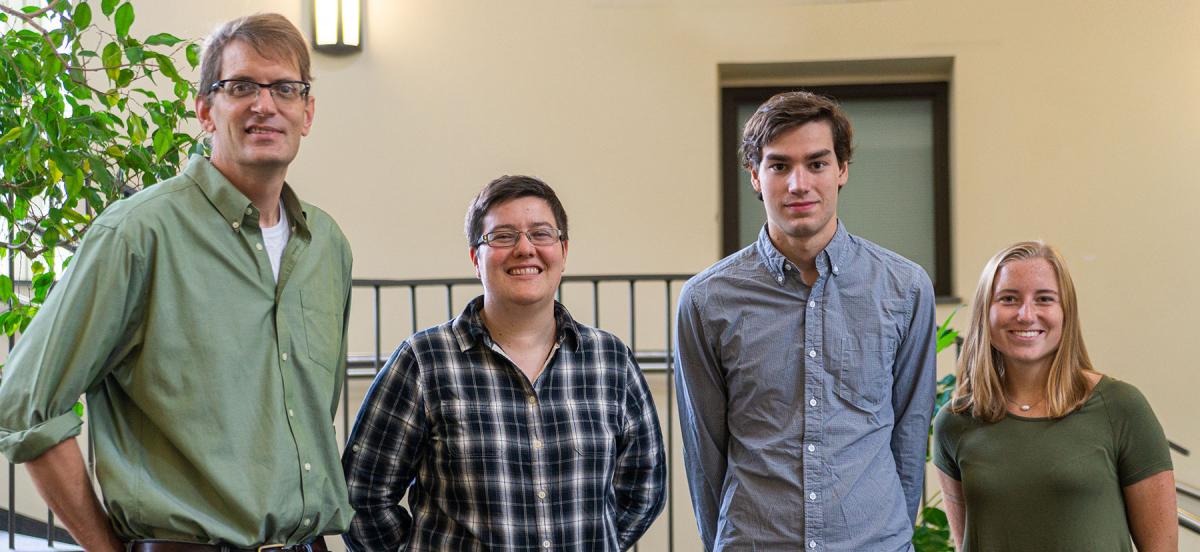
Some of the members of the research team behind the Nature paper: (from left) Professor of Chemistry Alexander Norquist, Assistant Professor of Computer Science Sorelle Friedler, Matt Danielson '21, and Allyson Lynch '21. Photo by Patrick Montero.
Details
The interdisciplinary faculty behind the “Dark Reactions Project” and eight of their students co-authored an article identifying previously unacknowledged human biases in chemical reaction data that impedes exploratory inorganic synthesis.
In 2016, a team of Haverford College scientists published the findings of their “Dark Reactions Project,” which mined failed experiments to predict new synthetic materials reactions, as a cover story in Nature. Now, three years later, that same team—Professor of Chemistry Alexander Norquist, Assistant Professor of Computer Science Sorelle Friedler, and Joshua Schrier, now of Fordham University—have returned to the pages of Nature with student co-authors to share the continuation of that research.
“In our ‘Dark Reactions’ paper, we showed that avoiding a failure bias enabled us to actually create a working model for crystallization,” said Norquist. “The literature is, of course, horribly biased against failure, and nearly all publicly available reaction data are of successes. Learning from these failed experiments was the focus of that paper. In the conception of this [new] work, we wondered if there were other biases that we should investigate and/or avoid.”
They found biases in both reactant and reaction condition selection. These biases were anthropogenic—or, of human origin—and they had been passed from scientists to machine-learning models that were being used to predict inorganic syntheses. By performing 548 randomly generated experiments, they showed that the popularity of reactants and the choices of reaction conditions were uncorrelated to the success of the reaction, and that randomly generated experiments better illustrated choices that that led to crystal formation. In other words, the machine-learning models they trained with their datasets better predicted reaction successes than those affected by the bias.
“We actually found an anthropogenic bias in scientist behavior that hinders our work,” said Norquist. “Scientists like to think of themselves as objective, but we're still humans who make biased decisions.”
Eight students co-authored the paper with Norquist, Friedler, and Schrier, including three recent grads who are all currently pursuing chemistry Ph.D.s. (Xiwen Jia '19 at Harvard, Oscar Huang '19 at Northwestern, and Alex Milder '18 at Ohio State.) Two current juniors—Matt Danielson and Allyson Lynch—were also part of the team, for which, Norquist said, students did much of the “actual work.” Danielson, for example, ran a number of the random reactions, generating the bulk of the new information for comparison against the historical, human-derived reactions, and Lynch performed statistical analysis of chemical databases, focusing on probabilistic distributions of usage in scientific literature, and developed Python code to automate the cleaning and processing of the data.
“I am very excited both to share what I worked on and the result, which I found to be very interesting,” said Lynch, an economics major from Ashburn, Va., of her first professional publication. “This was an incredible opportunity that I was afforded by Haverford to work with a professor on real research.”
“I find our work particularly interesting as it not only advances the field, but also offers a wider insight into human biases,” said Danielson, a chemistry major from Princeton, N.J.
In this age of “big data,” in which machine learning is used for so many things—from predicting purchases on Amazon to predicting chemical reactions in the lab—this research has wide-ranging implications. If people can be more aware of their inherent biases and work to avoid them, then the machine-learning algorithms made by those people can be less biased.
“Maybe the advantage of machine learning is not any ‘superhuman’ predictive capability, but that it’s consistent and is less likely to get stuck in the same cognitive biases that humans fall prey to,” wrote Norquist and Schrier in a blog post that accompanied the Nature paper. “If we want to realize that potential, then it is important to make sure that the training data doesn’t force algorithms to make the same mistakes as humans.”